Marketing Measurement Beyond ROI: Advanced KPIs for Modern Organizations
Apr 10, 2025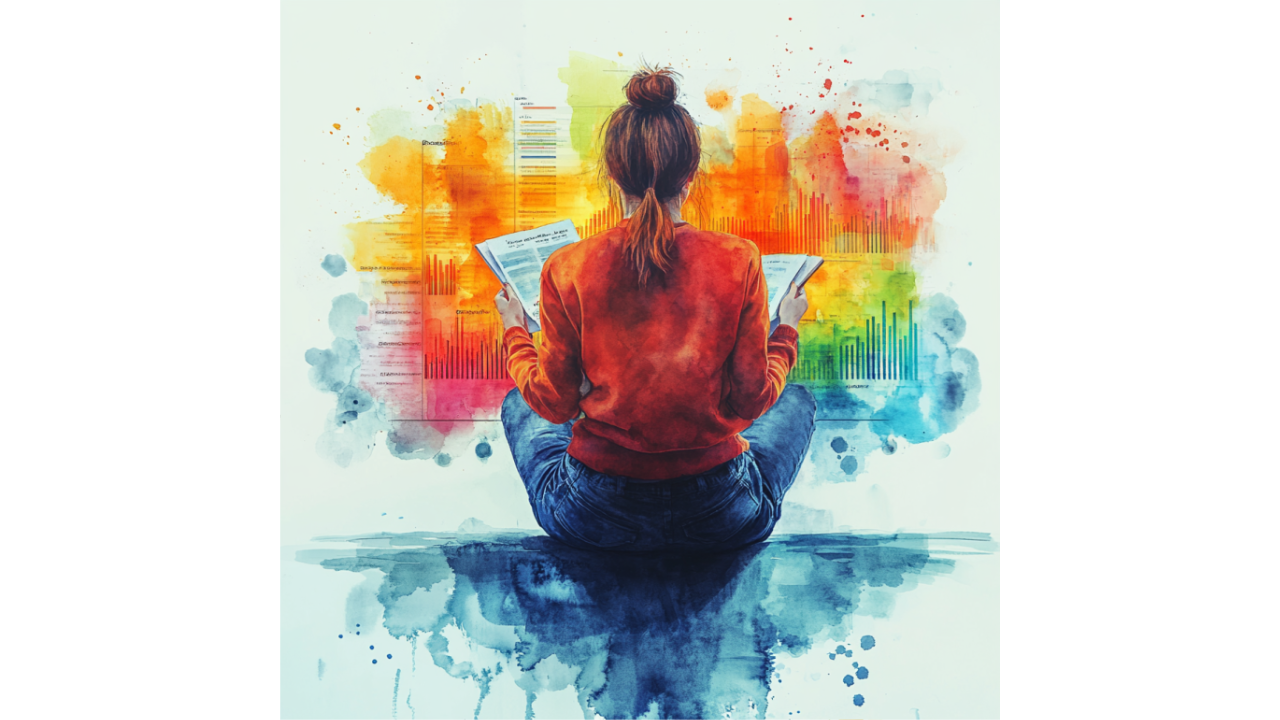
In the crowded conference room of a mid-sized tech company, the monthly marketing review takes a surprising turn. Instead of beginning with the traditional revenue attribution report, the CMO opens with a dashboard of metrics that would have been alien just three years ago: generative AI prompt effectiveness, multi-touch content attribution, voice search conversion paths, and chatbot resolution rates. Welcome to marketing measurement in 2025, where ROI remains important but insufficient on its own.
The Evolution of Marketing Measurement
The foundations of marketing measurement have shifted dramatically. While return on investment (ROI) remains a crucial north star metric, it fails to capture the complexity of modern customer journeys and the nuanced ways marketing technologies create value. Today's most sophisticated organizations are implementing expanded measurement frameworks that balance financial outcomes with leading indicators of future performance.
This shift recognizes that marketing's impact extends beyond immediate revenue generation to include building mental availability, creating customer loyalty, generating valuable first-party data, and creating competitive differentiation. Each of these contributions requires its own measurement approach.
The modern measurement stack typically includes four complementary layers:
- Financial metrics (ROI, ROAS, Customer Acquisition Cost)
- Customer journey metrics (engagement, conversion, attribution)
- Channel-specific performance indicators (email, social, search)
- Emerging technology metrics (AI, automation, conversational interfaces)
The organizations achieving the most accurate and actionable measurement combine these layers to tell a complete story about marketing's impact on both current performance and future potential.
Generative AI Engagement Metrics
As generative AI becomes integral to marketing experiences, new metrics have emerged to measure its effectiveness. These focus not just on traditional engagement but on the unique ways users interact with AI-powered content creation systems.
Prompt Effectiveness Rate (PER)
This metric measures the percentage of user prompts that result in successful AI-generated content. A successful generation is typically defined as one that requires minimal human editing (less than 20% modification) before use.
Calculation: (Number of prompts generating usable content / Total number of prompts) × 100
Current Benchmarks: The median PER across industries is approximately 65%, with high-performing teams achieving 80%+. B2B companies with specialized terminology typically see lower rates (50-60%) than consumer brands with more standardized language.
Example: An e-commerce company tracks that out of 500 product description prompts submitted to their AI content generator, 350 required minimal editing before publication, giving them a PER of 70%.
Content Acceleration Factor (CAF)
This measure quantifies how much faster content production becomes when using generative AI compared to traditional methods. It reflects the efficiency gains from AI implementation.
Calculation: (Average time for traditional content creation / Average time for AI-assisted content creation)
Current Benchmarks: Across content types, companies report CAFs ranging from 2.5× to 7×, with email and social copy seeing the highest acceleration (5-7×) and long-form content seeing more modest gains (2.5-4×).
Example: A marketing team that previously spent an average of 6 hours creating email campaigns now completes comparable campaigns in 1.2 hours using generative AI, yielding a CAF of 5.
Variation Effectiveness Ratio (VER)
This metric evaluates the performance differential between AI-generated content variations in A/B tests. It helps teams understand whether AI is effectively diversifying content approaches.
Calculation: (Performance spread between best and worst AI variations / Average performance) × 100
Current Benchmarks: Healthy VER values typically range from 20-40%. Values below 15% suggest the AI is creating variations that are too similar, while values above 45% may indicate inconsistent brand voice or quality.
Example: A SaaS company testing four AI-generated landing page headlines finds the best performer achieves a 12% conversion rate while the worst achieves 8%. With an average performance of 10%, this yields a VER of 40%.
Email Marketing Evolution Metrics
Email remains central to marketing programs, but its measurement has evolved to reflect changing user behaviors and platform limitations. With privacy changes affecting open rate reliability, new metrics have gained prominence.
Click-to-Open Ratio (CTOR)
This metric provides a more reliable engagement measure than open rates alone, showing the percentage of email openers who found content compelling enough to click.
Calculation: (Total clicks / Total opens) × 100
Current Benchmarks: Average CTOR across industries is 12-15%, with high-performing programs achieving 20%+. B2B newsletters typically see CTORs of 10-12%, while promotional emails with strong offers can reach 15-25%.
Example: If a newsletter had 5,000 opens and 750 clicks, the CTOR would be 15%.
Revenue Per Email (RPE)
This metric measures the average revenue generated by each email sent, providing a direct financial perspective on email performance.
Calculation: Total revenue attributed to email campaign / Number of emails delivered
Current Benchmarks: RPE varies dramatically by industry and email type. For e-commerce promotional emails, average RPE ranges from $0.08 to $0.15, while B2B nurture emails might generate $0.02 to $0.05 in pipeline value per send.
Example: An e-commerce flash sale email sent to 100,000 subscribers generated $12,000 in direct sales, yielding an RPE of $0.12.
List Engagement Depth (LED)
This metric looks beyond single campaigns to measure the percentage of your email list that has actively engaged (opened or clicked) within a specific timeframe.
Calculation: (Number of subscribers who engaged at least once in past 90 days / Total active subscribers) × 100
Current Benchmarks: Healthy email programs maintain LEDs of 40-60% over 90-day periods. Values below 30% may indicate list quality issues or content relevance problems.
Example: If 42,000 subscribers out of a total list of 80,000 have opened or clicked an email in the past 90 days, the LED would be 52.5%.
Chatbot and Conversational Interface Metrics
As chatbots and conversational interfaces become central to customer experience, specialized metrics have emerged to evaluate their performance.
Conversation Completion Rate (CCR)
This measure tracks the percentage of conversations where users successfully complete their intended goal without abandoning the interaction or requesting human intervention.
Calculation: (Number of conversations achieving user goal / Total conversations initiated) × 100
Current Benchmarks: Average CCR across industries is approximately 65%, with sophisticated implementations reaching 80%+. Customer service bots typically achieve 60-70%, while sales-oriented bots often range from 50-65%.
Example: If a support chatbot handled 1,200 customer inquiries and successfully resolved 840 without human intervention, the CCR would be 70%.
Containment Rate (CR)
This metric indicates how often a conversational interface can fully address user needs without transferring to a human agent.
Calculation: (Conversations fully handled by AI / Total conversations) × 100
Current Benchmarks: B2C companies average containment rates of 65-75% for customer service implementations, while B2B companies typically see slightly lower rates of 55-65% due to more complex inquiries.
Example: If out of 5,000 customer service conversations, 3,500 were fully handled by the chatbot without human intervention, the containment rate would be 70%.
Conversation Abandon Rate (CAR)
This measures the percentage of users who abandon a conversation before their issue is resolved or question answered.
Calculation: (Number of abandoned conversations / Total conversations initiated) × 100
Current Benchmarks: Best-in-class implementations maintain CARs below 15%. Rates between 15-25% are considered average, while rates above 30% indicate significant user experience issues.
Example: If 120 out of 1,000 chatbot conversations were abandoned before resolution, the CAR would be 12%.
Average Resolution Time (ART)
This metric tracks how quickly conversational interfaces resolve customer inquiries, measuring efficiency.
Calculation: Total time spent across all conversations / Number of resolved conversations
Current Benchmarks: Effective chatbots resolve simple inquiries in 2-4 minutes on average. Customer service implementations typically average 5-8 minutes across all conversation types, while sales-oriented applications may see longer times of 8-12 minutes due to more complex interactions.
Example: If a customer service chatbot spent a total of 14,400 minutes resolving 2,400 customer inquiries, the ART would be 6 minutes.
Attribution and Customer Journey Metrics
The increasing complexity of customer journeys has driven the development of more sophisticated attribution and journey analysis metrics.
Content Influence Score (CIS)
This metric quantifies how specific content assets influence conversion paths, even when they're not the final touchpoint before conversion.
Calculation: (Percentage of converting paths including the content asset) × (Average position in conversion path adjustment factor)
The adjustment factor weights early touchpoints less than later touchpoints, typically using values like 0.7 for awareness content, 0.9 for consideration content, and 1.0 for decision-stage content.
Current Benchmarks: Top-performing content typically achieves CIS values of 0.25-0.35, meaning they appear in 25-35% of conversion paths and have strong positioning. Average content scores between 0.10-0.20.
Example: If a whitepaper appears in 30% of conversion paths and typically serves as consideration content (0.9 adjustment), its CIS would be 0.27.
Multi-Touch Efficiency Ratio (MTER)
This metric evaluates the average number of marketing touchpoints required to generate a conversion, helping marketers understand journey efficiency.
Calculation: Total marketing touchpoints across all conversions / Number of conversions
Current Benchmarks: Efficiency varies by product complexity and price point. Simple consumer products typically convert in 3-5 touches, while complex B2B solutions may require 8-12 touches on average.
Example: If analysis shows 10,000 total marketing touchpoints contributed to 2,000 conversions, the MTER would be 5.
Path Diversity Index (PDI)
This metric measures how many distinct conversion paths contribute significantly to your marketing results, indicating resilience and channel diversity.
Calculation: Number of distinct paths contributing to at least 1% of conversions
Current Benchmarks: Mature omnichannel programs typically have PDIs of 15-25, indicating diverse, resilient marketing ecosystems. Values below 10 suggest over-reliance on a few dominant paths.
Example: If analysis shows 17 different conversion paths each contributing at least 1% of total conversions, the PDI would be 17.
Marketing Technology Performance Metrics
The expanding marketing technology stack requires its own set of performance metrics to ensure these systems are delivering value.
Marketing Automation Efficiency (MAE)
This metric compares the time saved through marketing automation to the time invested in maintaining and optimizing these systems.
Calculation: (Estimated hours saved through automation per month / Hours spent maintaining automation systems per month)
Current Benchmarks: Mature marketing automation implementations typically achieve MAE ratios of 5:1 to 8:1. Ratios below 3:1 may indicate overly complex or inefficient automation.
Example: If a company's marketing automation saves approximately 160 hours of manual work monthly while requiring 25 hours of maintenance and optimization, the MAE would be 6.4.
Technology Adoption Index (TAI)
This measures how effectively marketing team members are utilizing available marketing technologies.
Calculation: Average of (Number of features used / Total available features) across all major marketing platforms
Current Benchmarks: Top marketing organizations achieve TAIs of 65-75%, while average implementations typically utilize only 40-50% of available functionality.
Example: If a team uses 24 out of 40 features in their CRM, 18 out of 30 in their marketing automation platform, and 12 out of 20 in their analytics suite, their TAI would be 60%.
Tech Stack Integration Score (TSIS)
This metric evaluates how seamlessly different marketing technologies share data and functionality.
Calculation: (Number of bidirectional integrations between systems / Maximum possible integrations) × 100
Current Benchmarks: Well-integrated tech stacks achieve scores of 80%+, meaning most systems share data bidirectionally. Average marketing organizations typically score 50-65%.
Example: If a company has 5 core marketing systems that could have a maximum of 10 bidirectional integrations, but only 7 are fully implemented, their TSIS would be 70%.
Applied Metrics Framework: Bringing It All Together
The most advanced marketing organizations integrate these metrics into cohesive measurement frameworks that tell a complete story about marketing performance. Rather than viewing these measures in isolation, they create dashboards that show how metrics relate to each other and influence business outcomes.
One effective approach is the pyramid framework:
Foundation Metrics (Technology and Operations)
- Marketing Automation Efficiency
- Technology Adoption Index
- Tech Stack Integration Score
- Content Acceleration Factor
Middle Tier (Channel and Journey Performance)
- Conversation Completion Rate
- Click-to-Open Ratio
- Path Diversity Index
- Prompt Effectiveness Rate
Top Tier (Business Impact)
- Revenue Per Email
- Content Influence Score
- Traditional ROI and ROAS metrics
- Customer Lifetime Value
This hierarchical approach helps organizations understand how foundational marketing technology performance influences channel and journey effectiveness, which ultimately drives business results.
Implementation Considerations
When implementing these advanced metrics, several best practices can help ensure success:
- Start with clear business objectives. Select metrics that align with your organization's strategic priorities rather than tracking everything possible.
- Build measurement maturity gradually. Begin with foundational metrics before moving to more sophisticated measures like Content Influence Score or Path Diversity Index.
- Ensure data quality and consistency. Advanced metrics require reliable data from multiple systems. Invest in data governance and integration before attempting complex measurements.
- Establish benchmarks and targets. Use industry benchmarks as starting points, but develop organization-specific targets based on your unique situation and historical performance.
- Create visualization dashboards. Present metrics in context using visualization tools that show relationships between different measures and highlight trends over time.
The organizations achieving the most accurate and actionable measurement recognize that metrics serve not just to report performance but to guide strategy. They create measurement systems that highlight opportunities, predict outcomes, and recommend actions based on real-time data.
Measurement as Competitive Advantage
As marketing technology and customer behavior continue to evolve, measurement capabilities have become a genuine competitive advantage. Organizations that can accurately track performance across traditional and emerging channels gain an edge in optimizing budget allocation, improving customer experiences, and accelerating growth.
The metrics outlined here represent the current state of advanced marketing measurement, but the field continues to evolve rapidly. Forward-thinking marketers are already exploring new measures for emerging channels like augmented reality, virtual product experiences, and decentralized commerce platforms.
What remains constant is the need to balance immediate performance indicators with metrics that signal future potential. The most valuable measurement frameworks capture both the "what" and the "why" of marketing performance, providing both validation of past decisions and guidance for future strategies.
Join us at ACE to master these advanced marketing measurement approaches. Our Data-Driven Marketing course will equip you with the frameworks, tools, and techniques to implement these metrics in your organization and transform measurement from a reporting function to a strategic advantage.
GET ON OUR NEWSLETTER LIST
Sign up for new content drops and fresh ideas.